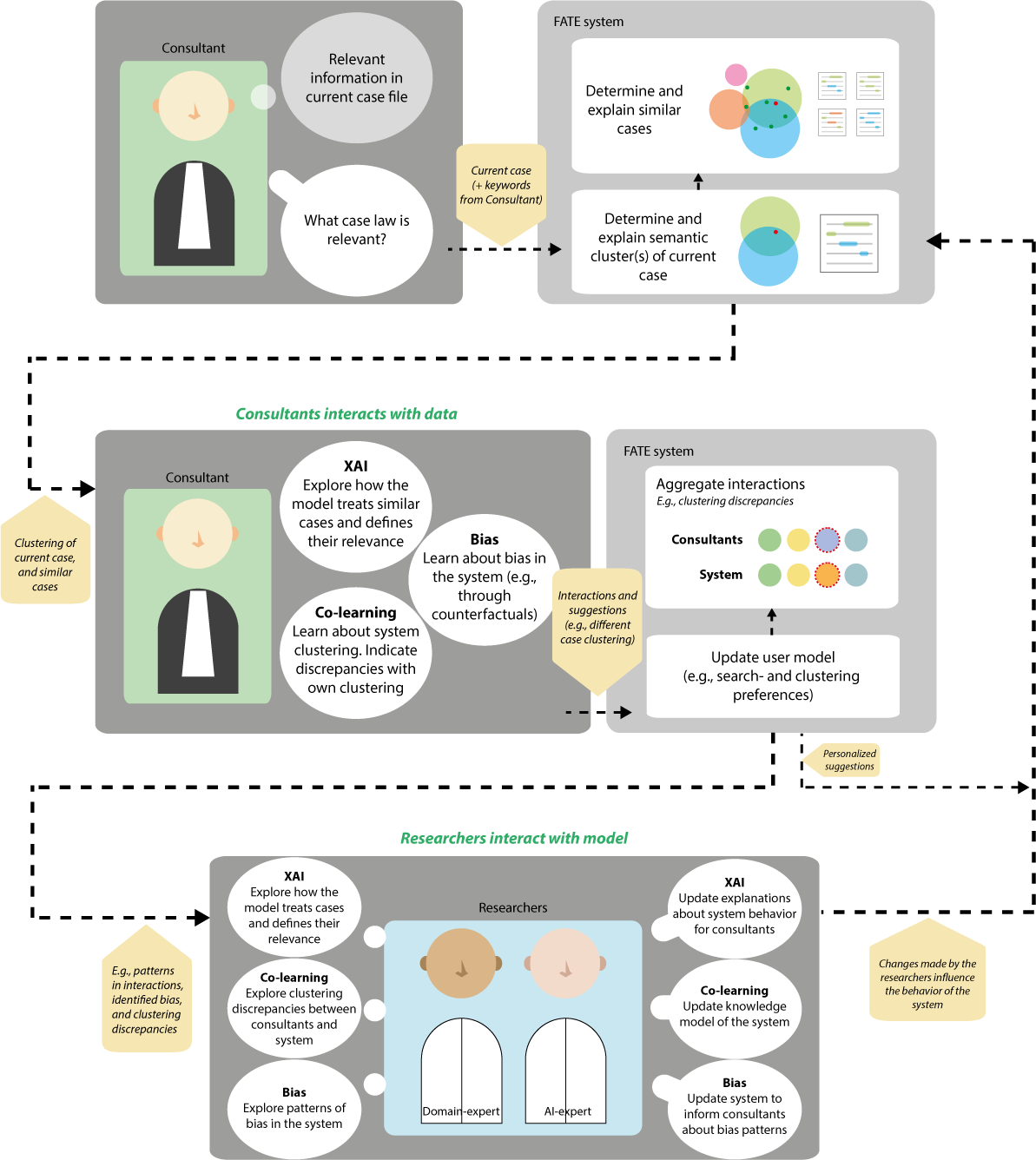
More and more, virtual agents and social robots will enter our working and leisure environments with increasing adaptation and learning capabilities. This provides new opportunities to develop hybrid (human-AI) intelligence, comprising new ways of collaboration. Both humans and AI continuously learn over time. This includes learning the tasks, but they also learn from and about each other. What the nature of such evolving Hybrid Intelligence (HI) will be is yet difficult to predict, and will most likely continue to change over time. HI can be characterized as a human-AI system that co-evolves through mutual adaptation and learning, which may occur implicitly, as well as explicitly. The design of hybrid intelligence should elicit the behavior from partners that supports mutual adaptation and learning. Humans should be enabled and encouraged to view AI-agents as dynamic and learning partners. At the same time, AI-agents need to have capabilities to develop an understanding of the partners and the team. Together, this demands a new type of team (“a new breed”) that learns to collaborate in a symbiotic fashion. A core research question is then: How to design such new human-AI systems?
The literature uses different terms for the ongoing development of a hybrid team: co-adaptation, co-learning and co-evolution. While these terms are often used interchangeably, they can be distinguished on three dimensions, namely: 1) the time-frame in which the developments takes place (shorter for co-adaptation, longer for co-evolution); 2) the persistence of the acquired knowledge and behaviors over time and across contexts (persistence is strongest in co-learning and co-evolution); and 3) the role of intention initiating the development (co-learning is much more a product of partners’ intentions than co-adaptation and co-evolution are) (van Zoelen et al., 2021). Understanding how these processes shape a team’s development is of critical importance when designing an HI-system, because then the proper interactions can be elicited that trigger the intended processes.
To give an example: the FATE project (de Greeff et al., 2021) developed AI functionalities for the design of a hybrid system providing support in making judicial decisions. Different users were considered, for example, a researcher, a lawyer, and a defendant, each with their own requirements. It has been explored how AI functionalities like bias detection, secure learning, explainable AI and co-learning affect and can be of use to these different types of users. Vital questions in these efforts are how each type of user contributes to the HI-system, as well as how we can ensure that every actor in the system can learn from the knowledge of the others. The outcome can be seen in Figure 1. It illustrates how different users interact with the AI on different levels, constantly providing their feedback to improve the performance of the HI-system. Human-AI interactive systems are often viewed as dyadic interaction between AI and a single user (e.g., an autonomous vehicle). However, such systems involve more people too, especially when the AI is a data-driven system that uses data from many different users. This makes processes like co-learning and co-evolution complex.
In this workshop, participants will address and discuss the design and evaluation of HI-systems, aiming at advanced performance and well-being. This will include, for example, insights from evolutionary processes such as symbiosis and habituation, discussed at the level of hybrid teams and communities. Furthermore, the application of design patterns for the iterative design of HI-systems will be explored.
The workshop aims to connect the new generation of researchers on the design of Hybrid Intelligence and provides an opportunity to learn from each other by sharing research questions and experiences. Attendees will also work in interdisciplinary groups on different research scenarios.
Relevant References
Damiano, L., & Dumouchel, P. (2018). Anthropomorphism in human–robot co-evolution. Frontiers in psychology, 9, 468.
Dengel, A., Devillers, L., & Schaal, L. M. (2021). Augmented Human and Human-Machine Co-evolution: Efficiency and Ethics. In Reflections on Artificial Intelligence for Humanity (pp. 203-227). Springer, Cham.
De Greeff, J., de Boer, M. H., Hillerström, F. H., Bomhof, F., Jorritsma, W., & Neerincx, M. A. (2021). The FATE System: FAir, Transparent and Explainable Decision Making. In AAAI Spring Symposium: Combining Machine Learning with Knowledge Engineering.
Lee, E. A. (2020). The Coevolution: The Entwined Futures of Humans and Machines. Mit Press.
Neerincx, M. A., Van Vught, W., Blanson Henkemans, O., Oleari, E., Broekens, J., Peters, R., ... & Bierman, B. (2019). Socio-cognitive engineering of a robotic partner for child's diabetes self-management. Frontiers in Robotics and AI, 118. https://doi.org/10.3389/frobt.2019.00118.
Van der Waa, J., van Diggelen, J., Cavalcante Siebert, L., Neerincx, M., & Jonker, C. (2020). Allocation of moral decision-making in human-agent teams: a pattern approach. In International Conference on Human-Computer Interaction (pp. 203-220). Springer, Cham.
Van Zoelen, E. M., Van Den Bosch, K., & Neerincx, M. (2021). Becoming team members: identifying interaction patterns of mutual adaptation for human-robot co-learning. Frontiers in Robotics and AI, 8.